I wrote a simple simulation code in two different versions (Python, HOC).
I'm curious as to why the execution results are different.
The content of the simulation code is to provide a step current input to a single compartment cell model and to draw a graph of the membrane potential.
The codes below are the two versions I wrote and the results of their execution.
(the spike width is widened in Python ver.)
Any ideas on this problem would be really appreciated!
Sincerely,
Kisung Shin
HOC version
Code: Select all
// load nrngui
load_file("nrngui.hoc")
// define cell template
begintemplate Cell
public soma
create soma
proc init(){
soma {
diam = 12.6157
L = 12.6157
nseg = 5
cm = 1
Ra = 100
insert pas
e_pas = -65
g_pas = 0.001
insert hh2
gnabar_hh2 = 0.12
gkbar_hh2 = 0.036
vtraub_hh2 = -64.2
insert im
gkbar_im = 0.000001
ena = 50
ek = -90
}
}
endtemplate Cell
// make cell object
objref cell
cell = new Cell()
// make step current input
objref stim
stim = new IClamp(0.5)
stim.del = 1100
stim.dur = 2
stim.amp = 1
// set graph of voltage trace
objref g
g = new Graph()
g.addexpr("cell.soma.v(0.5)")
g.size(1000, 1300, -100, 50)
graphList[0].append(g)
// set simulation parameter & run
v_init = -65
dt = 0.2
tstop = 2000
celsius = 30
run()
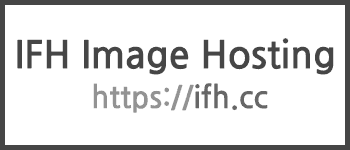
Python version
Code: Select all
# import library
from neuron import h, gui
import matplotlib.pyplot as plt
# define cell class
class Cell():
def __init__(self):
super().__init__()
self.soma = h.Section(name = 'soma', cell = self)
self.soma.diam = 12.6157
self.soma.L = 12.6157
self.soma.nseg = 5
self.soma.cm = 1
self.soma.Ra = 100
self.soma.insert('pas')
for seg in self.soma:
seg.pas.e = -65
seg.pas.g = 0.001
self.soma.insert('hh2')
for seg in self.soma:
seg.hh2.gnabar = 0.12
seg.hh2.gkbar = 0.036
seg.hh2.vtraub = -64.2
self.soma.insert('im')
for seg in self.soma:
seg.im.gkbar = 0.000001
self.soma.ena = 50
self.soma.ek = -90
# make cell object
cell = Cell()
# make step current input
stim = h.IClamp(cell.soma(0.5))
stim.delay = 1100
stim.dur = 2
stim.amp = 1
# set recorder for graph
tvec = h.Vector().record(h._ref_t)
vvec = h.Vector().record(cell.soma(0.5)._ref_v)
# set simulation parameter & run
h.finitialize(-65)
h.dt = 0.2
h.continuerun(2000)
h.celsius = 30
# plot voltage trace
plt.figure()
plt.plot(tvec, vvec)
plt.xlim(1000, 1300)
plt.ylim(-100, 50)
plt.show()
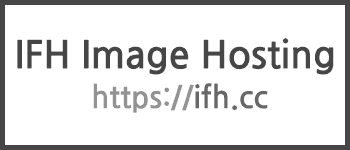
hh2.mod
Code: Select all
TITLE Hippocampal HH channels
:
: Fast Na+ and K+ currents responsible for action potentials
: Iterative equations
:
: Equations modified by Traub, for Hippocampal Pyramidal cells, in:
: Traub & Miles, Neuronal Networks of the Hippocampus, Cambridge, 1991
:
: range variable vtraub adjust threshold
:
: Written by Alain Destexhe, Salk Institute, Aug 1992
:
: Modified Oct 96 for compatibility with Windows: trap low values of arguments
:
: Modifications by Arthur Houweling for use in MyFirstNEURON
: Modifications by Paulo Aguiar: vh changed from 5 to 6 - NOT ANYMORE: vh=5 as originally set
INDEPENDENT {t FROM 0 TO 1 WITH 1 (ms)}
NEURON {
SUFFIX hh2
USEION na READ ena WRITE ina
USEION k READ ek WRITE ik
RANGE gnabar, gkbar, vtraub
RANGE m_inf, h_inf, n_inf
RANGE tau_m, tau_h, tau_n
RANGE m_exp, h_exp, n_exp
RANGE ik, ina
}
UNITS {
(mA) = (milliamp)
(mV) = (millivolt)
}
PARAMETER {
gnabar = .1 (mho/cm2)
gkbar = .06 (mho/cm2)
ena (mV)
ek (mV)
celsius (degC)
dt (ms)
v (mV)
vtraub = -55 (mV) : adjusts threshold
}
STATE {
m h n
}
ASSIGNED {
ina (mA/cm2)
ik (mA/cm2)
il (mA/cm2)
m_inf
h_inf
n_inf
tau_m
tau_h
tau_n
m_exp
h_exp
n_exp
tadj
}
BREAKPOINT {
SOLVE states METHOD cnexp
ina = gnabar * m*m*m*h * (v - ena)
ik = gkbar * n*n*n*n * (v - ek)
}
DERIVATIVE states { : use this for exact Hodgkin-Huxley equations
evaluate_fct(v)
m' = (m_inf - m) / tau_m
h' = (h_inf - h) / tau_h
n' = (n_inf - n) / tau_n
}
:PROCEDURE states() { : this discretized form is more stable
: evaluate_fct(v)
: m = m + m_exp * (m_inf - m)
: h = h + h_exp * (h_inf - h)
: n = n + n_exp * (n_inf - n)
: VERBATIM
: return 0;
: ENDVERBATIM
:}
UNITSOFF
INITIAL {
:
: Q10 was assumed to be 3 for both currents
:
tadj = 3.0 ^ ((celsius-36)/ 10 )
evaluate_fct(v)
m= m_inf
h= h_inf
n= n_inf
}
PROCEDURE evaluate_fct(v(mV)) { LOCAL a,b,v2, vh
v2 = v - vtraub : convert to traub convention
vh = 0 : Original Value = 5
: a = 0.32 * (13-v2) / ( exp((13-v2)/4) - 1)
a = 0.32 * vtrap(13-v2, 4)
: b = 0.28 * (v2-40) / ( exp((v2-40)/5) - 1)
b = 0.28 * vtrap(v2-40, 5)
tau_m = 1 / (a + b) / tadj
m_inf = a / (a + b)
:a = 0.128 * exp((17-v2)/18)
:b = 4 / ( 1 + exp((40-v2)/5) )
:tau_h = 1 / (a + b) / tadj
:h_inf = a / (a + b)
a = 0.128 * exp((17-v2-vh)/18)
b = 4 / ( 1 + exp((40-v2-vh)/5) )
tau_h = 1 / (a + b) / tadj
h_inf = a / (a + b)
: a = 0.032 * (15-v2) / ( exp((15-v2)/5) - 1)
a = 0.032 * vtrap(15-v2, 5)
b = 0.5 * Exp((10-v2)/40)
tau_n = 1 / (a + b) / tadj
n_inf = a / (a + b)
m_exp = 1 - Exp(-dt/tau_m)
h_exp = 1 - Exp(-dt/tau_h)
n_exp = 1 - Exp(-dt/tau_n)
}
FUNCTION vtrap(x,y) {
if (fabs(x/y) < 1e-6) {
vtrap = y*(1 - x/y/2)
}else{
vtrap = x/(Exp(x/y)-1)
}
}
FUNCTION Exp(x) {
if (x < -100) {
Exp = 0
}else{
Exp = exp(x)
}
}
Code: Select all
TITLE Cortical M current
:
: M-current, responsible for the adaptation of firing rate and the
: afterhyperpolarization (AHP) of cortical pyramidal cells
:
: First-order model described by hodgkin-Hyxley like equations.
: K+ current, activated by depolarization, noninactivating.
:
: Model taken from Yamada, W.M., Koch, C. and Adams, P.R. Multiple
: channels and calcium dynamics. In: Methods in Neuronal Modeling,
: edited by C. Koch and I. Segev, MIT press, 1989, p 97-134.
:
: See also: McCormick, D.A., Wang, Z. and Huguenard, J. Neurotransmitter
: control of neocortical neuronal activity and excitability.
: Cerebral Cortex 3: 387-398, 1993.
:
: Written by Alain Destexhe, Laval University, 1995
:
INDEPENDENT {t FROM 0 TO 1 WITH 1 (ms)}
NEURON {
SUFFIX im
USEION k READ ek WRITE ik
RANGE gkbar, ik, m_inf, tau_m
GLOBAL taumax
}
UNITS {
(mA) = (milliamp)
(mV) = (millivolt)
}
PARAMETER {
v (mV)
celsius = 36 (degC)
ek (mV)
gkbar = 1e-6 (mho/cm2)
taumax = 1000 (ms) : peak value of tau
}
STATE {
m
}
ASSIGNED {
ik (mA/cm2)
m_inf
tau_m (ms)
tau_peak (ms)
tadj
}
BREAKPOINT {
SOLVE states METHOD cnexp
ik = gkbar * m * (v - ek)
}
DERIVATIVE states {
evaluate_fct(v)
m' = (m_inf - m) / tau_m
}
UNITSOFF
INITIAL {
evaluate_fct(v)
m = 0
:
: The Q10 value is assumed to be 2.3
:
tadj = 2.3 ^ ((celsius-36)/10)
tau_peak = taumax / tadj
}
PROCEDURE evaluate_fct(v(mV)) {
m_inf = 1 / ( 1 + exptable(-(v+35)/10) )
tau_m = tau_peak / ( 3.3 * exptable((v+35)/20) + exptable(-(v+35)/20) )
}
UNITSON
FUNCTION exptable(x) {
TABLE FROM -25 TO 25 WITH 10000
if ((x > -25) && (x < 25)) {
exptable = exp(x)
} else {
exptable = 0.
}
}
https://senselab.med.yale.edu/ModelDB/S ... %2fHH2.mod
https://senselab.med.yale.edu/ModelDB/S ... x%2fIM.mod